Invited Talks
Benchmarks are the keystone that hold the machine learning community together. Growing as a research paradigm since the 1980s, there's much we've done with them, but little we know about them. In this talk, I will trace the rudiments of an emerging science of benchmarks through selected empirical and theoretical observations. Specifically, we'll discuss the role of annotator errors, external validity of model rankings, and the promise of multi-task benchmarks. The results in each case challenge conventional wisdom and underscore the benefits of developing a science of benchmarks.
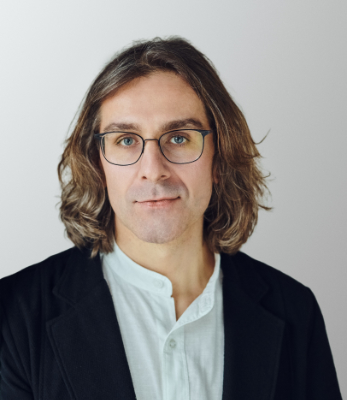
Moritz Hardt
Hardt is a director at the Max Planck Institute for Intelligent Systems, Tübingen. Previously, he was Associate Professor for Electrical Engineering and Computer Sciences at the University of California, Berkeley. His research contributes to the scientific foundations of machine learning and algorithmic decision making with a focus on social questions. He co-authored Fairness and Machine Learning: Limitations and Opportunities (MIT Press) and Patterns, Predictions, and Actions: Foundations of Machine Learning (Princeton University Press).
After decades of steady progress and occasional setbacks, the field of AI now finds itself at an inflection point. AI products have exploded into the mainstream, we've yet to hit the ceiling of scaling dividends, and the community is asking itself what comes next. In this talk, Raia will draw on her 20 years experience as an AI researcher and AI leader to examine how our assumptions about the path to Artificial General Intelligence (AGI) have evolved over time, and to explore the unexpected truths that have emerged along the way. From reinforcement learning to distributed architectures and the potential of neural networks to revolutionize scientific domains, Raia argues that embracing lessons from the past offers valuable insights for AI's future research roadmap.
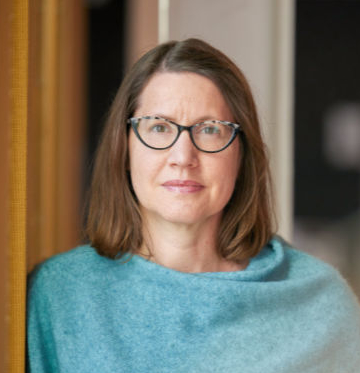
Raia Hadsell
I am a Senior Director of Research and Robotics at DeepMind. I joined DeepMind in 2014 to pursue new solutions for artificial general intelligence. Currently, I oversee the strategy for DeepMind’s exploratory research efforts, leading teams exploring new innovations in AI that might address the open questions that today's techniques cannot answer.
Before joining DeepMind in early 2014, I had found my way into AI research obliquely. After an undergraduate degree in religion and philosophy from Reed College, I veered off-course (on-course?) and became a computer scientist. My PhD with Yann LeCun, at NYU, focused on machine learning using Siamese neural nets (often called a 'triplet loss' today), face recognition algorithms, and on deep learning for mobile robots in the wild. My thesis, 'Learning Long-range vision for offroad robots', was awarded the Outstanding Dissertation award in 2009. I spent a post-doc at CMU Robotics Institute, working with Drew Bagnell and Martial Hebert, and then became a research scientist at SRI International, at the Vision and Robotics group in Princeton, NJ.
After joining DeepMind, then a small 50-person startup that had just been acquired by Google, my research focused on a number of fundamental challenges in AGI, including continual and transfer learning, deep reinforcement learning for robotics and control problems, and neural models of navigation (see publications). I have proposed neural approaches such as policy distillation, progressive nets, and elastic weight consolidation to solve the problem of catastrophic forgetting.
In the broader AI community, I am founder and Editor-in-Chief of a new open journal, TMLR. I sit on the executive board of CoRL, am a fellow of the European Lab on Learning Systems (ELLIS), and a founding organizer of NAISys (Neuroscience for AI Systems). I also serves as a CIFAR advisor and have previously sat on the Executive Board for WiML (Women in Machine Learning).
Large language models have substantially advanced the state of the art in various AI tasks, such as natural language understanding and text generation, and image processing, multimodal modeling. In this talk, we will first introduce the development of AI in the past decades, in particular from the angle of China. We will also talk about the opportunities, challenges, and risks of AGI in the future. In the second part of the talk, we will use ChatGLM, an alternative but open-sourced model to ChatGPT, as an example to explain our understanding and insights derived during the implementation of the model.
Jie Tang
This talk will cover fundamental legal principles all AI researchers should understand. This talk will explore why legislators are looking at new laws specifically for AI and the goals they want to accomplish with those laws. It will also cover legal risks related to using training datasets, understanding dataset licenses, and options for licensing models in an open fashion.
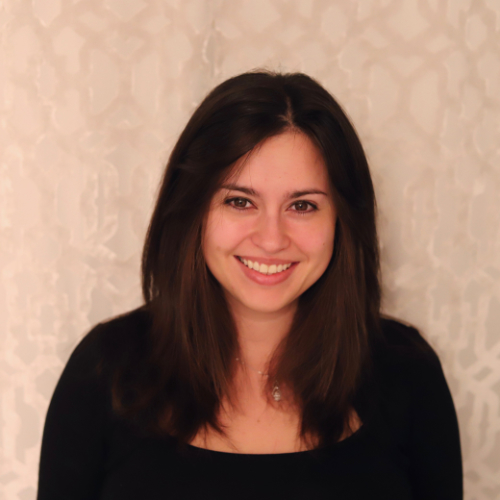
Kate Downing
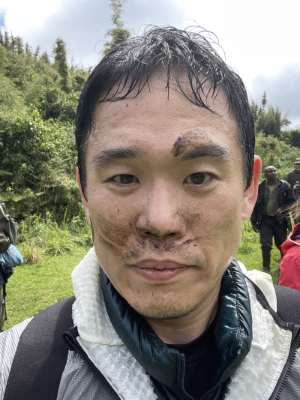
Kyunghyun Cho
Kyunghyun Cho is a professor of computer science and data science at New York University and a senior director of frontier research at the Prescient Design team within Genentech Research & Early Development (gRED). He is also a CIFAR Fellow of Learning in Machines & Brains and an Associate Member of the National Academy of Engineering of Korea. He served as a (co-)Program Chair of ICLR 2020, NeurIPS 2022 and ICML 2022. He is also a founding co-Editor-in-Chief of the Transactions on Machine Learning Research (TMLR). He was a research scientist at Facebook AI Research from June 2017 to May 2020 and a postdoctoral fellow at University of Montreal until Summer 2015 under the supervision of Prof. Yoshua Bengio, after receiving MSc and PhD degrees from Aalto University April 2011 and April 2014, respectively, under the supervision of Prof. Juha Karhunen, Dr. Tapani Raiko and Dr. Alexander Ilin. He received the Samsung Ho-Am Prize in Engineering in 2021. He tries his best to find a balance among machine learning, natural language processing, and life, but almost always fails to do so.